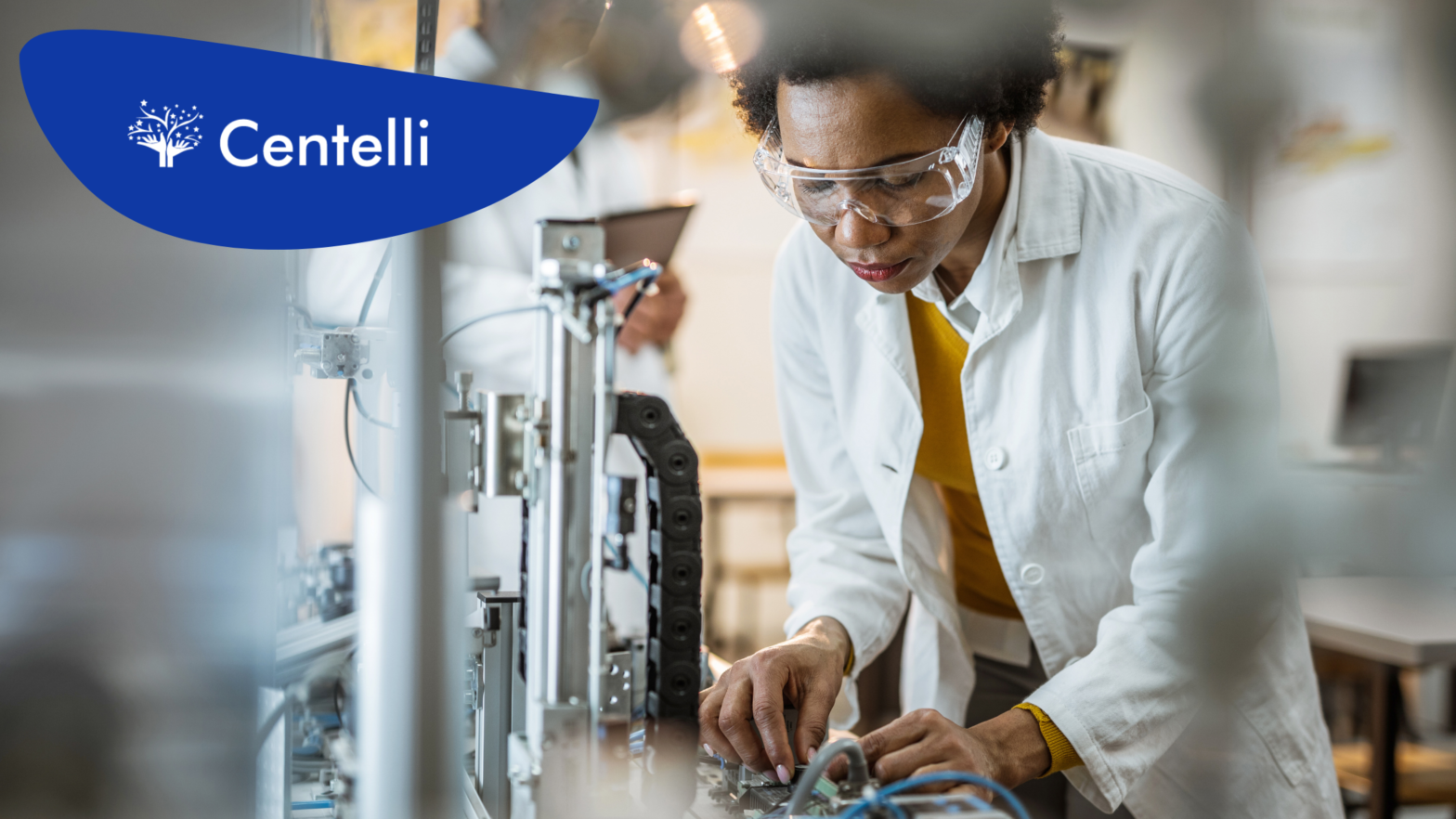
- Digital Workers
- Written By Harriet Kotvics
How Machine Learning and Intelligent Automation Combat Antibiotic Resistance
22-Mar-2024 . 3 min read
At Centelli, we’re well aware of the impact that AI and Automation can have. We work with various Healthcare businesses to improve performance, patient care, and efficiencies. This is mostly done by assisting in back-office and front-office functions, but we’re also acutely aware of how the same technology can help much more broadly to improve treatments and health outcomes. So, how exactly does Machine Learning and Intelligent Automation combat antibiotic resistance?
Tuberculosis (TB), caused by bacteria known as Mycobacterium tuberculosis, is a prime example of a disease where antibiotic resistance poses a significant threat to effective treatment. However, integrating intelligent automation and machine learning offers a groundbreaking approach to tackling this global health issue.
Recent advancements highlighted in a study conducted at the University of California, San Diego, demonstrate the power of machine learning in identifying antibiotic-resistance genes within TB-causing bacteria[1]. This breakthrough highlights technology’s potential to enhance medical research and holds promise for transforming how we combat infectious diseases.
Intelligent automation and machine learning algorithms empower researchers to sift through vast amounts of genetic data with unprecedented speed and precision[2]. By analysing the genetic makeup of TB bacteria, these algorithms can swiftly identify genetic markers associated with antibiotic resistance. This newfound efficiency accelerates the discovery process, enabling scientists to pinpoint potential targets for novel treatments more rapidly than ever before.
The implications of this technological synergy extend far beyond TB treatment. Intelligent automation and machine learning can potentially revolutionise healthcare globally.
Here’s how:
- Personalised Medicine: By leveraging machine learning algorithms, healthcare providers can tailor treatment plans to individual patients based on their genetic profiles[3]. This customised approach enhances treatment efficacy while minimising adverse effects, ultimately improving patient outcomes.
- Early Disease Detection: Intelligent automation facilitates the analysis of vast datasets from various sources, enabling early detection of diseases and health risks. Machine learning algorithms can identify subtle patterns indicative of disease onset[4], allowing for timely intervention and preventive measures.
- Drug Discovery and Development: Integrating machine learning in drug discovery expedites the identification of potential therapeutic compounds and accelerates the development process. By analysing complex biological data, researchers can more accurately predict the efficacy and safety of candidate drugs, streamlining the path to novel treatments.
- Healthcare Accessibility: Intelligent automation enhances the efficiency of healthcare delivery systems, making quality care more accessible to underserved populations. From telemedicine platforms to AI-powered diagnostics, technology-driven solutions bridge gaps in healthcare access, ensuring that everyone can benefit from advancements in medical science.
Intelligent Automation and machine learning are beacons of innovation, illuminating pathways to better health outcomes for all. By integrating these techniques, our clients drive huge efficiencies, massively speed up patient treatment, and ultimately make their operations much more efficient. However, AI and automation can also confront challenges like antibiotic resistance head-on, ushering in a new phase of personalised, data-driven medicine that can revolutionise lives worldwide.
[1] Step-by-step account of HIV-1 infection | The Scientist Magazine®. https://www.the-scientist.com/research-round-up/step-by-step-account-of-hiv-1-infection-54571
[2] The Sainsbury Laboratory | Pikobodies: A new hope for made-to-order…. https://www.tsl.ac.uk/news/pikobodies-a-new-hope-for-made-to-order-plant-disease-resistance
[3] ] AYUSH collaboration with ICMR for scientific validation – Rau’s IAS. https://compass.rauias.com/current-affairs/ayush-collaboration-with-icmr-for-scientific-validation/
[4] Machine Learning in Healthcare: Transforming Medical Diagnostics – IABAC. https://iabac.org/blog/machine-learning-in-healthcare-transforming-medical-diagnostics